It’s popular today to talk about machine learning. Artificial Intelligence is encompassing almost every work field there is. A “Learned Machine” is your ally at work and a valuable assistant. From tailored recommendations to seamless fraud detection, machine learning is driving efficiency and personalization across various sectors. Learn about machine learning applications that are useful in everyday life in this article.
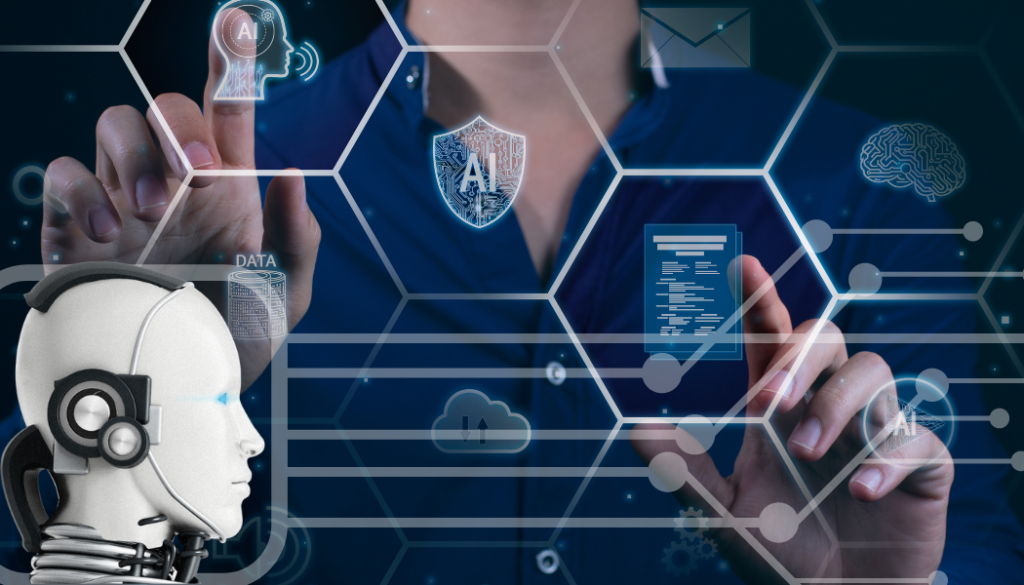
The Inner Workings of Machine Learning
At the basis of ML lies an algorithm that is trying to decipher patterns to make predictions and decisions without explicit programming. Imagine it as a detective sifting through clues to crack a case. Take targeted ads, for instance. They seem to know your every interest and preference, unless they are showing you cauches that you have once search a month ago to help your friend.
Examples of Machine Learning Applications in Everyday Life
There are many different applications of ML that can make your life easier. You can enhance your own performance with it or experience it on the internet while browsing. Some of the applications are:
1. Personalized Content Recommendations
Streaming platforms like Netflix analyze your viewing history to suggest shows and movies you’re likely to enjoy, creating a personalized entertainment experience. This algorithm falls apart shortly, though, if several people with different interests use the same account.
2. Efficient Auto-correct Functionality
Ever wondered how your phone knows exactly what you meant to type, even with typos? That’s machine learning at work, continuously refining its predictions based on your typing habits. You wanting to type something new but the Auto-correction makes it something wrong? That’s machine learning as well.
3. Enhanced Fraud Detection
Banks employ machine learning algorithms to detect suspicious activities and prevent fraudulent transactions, safeguarding your finances in real-time.
4. Virtual Personal Assistants
Virtual assistants such as Siri and Alexa utilize machine learning to understand and respond to user queries, providing assistance tailored to individual preferences.
5. Seamless Traffic Prediction
Apps like Google Maps leverage machine learning to analyze traffic data and suggest optimal routes, helping you navigate through congested roads efficiently.
6. Social Network Recommendations
Social media platforms utilize machine learning algorithms to suggest potential connections based on factors like mutual friends, interests, and location. And like any recommendation, take it with a grain of salt.
7. Robust Spam Filters
Email providers utilize machine learning to filter out spam and phishing emails, ensuring your inbox remains clutter-free and secure. With that said, only keeping the filters updated doesn’t guarantee your safety. New undetected threats appear regularly.
8. Smart Wearable Devices
From fitness trackers to smartwatches, wearable devices leverage machine learning to track your activities, monitor health metrics, and provide personalized insights. Yet, again. Privacy concerns are always there.
9. Facial Recognition Technology
Applications like the Lensa AI app utilize machine learning algorithms to analyze biometric data and identify individuals, enhancing security measures and user authentication. Unless you have a twin, that is.
10. Personalized Music Recommendations
Streaming services like Spotify analyze your listening habits to curate personalized playlists tailored to your musical preferences. Like anything personalized, if interests are diverse it’s a rather useless feature.
Types of Machine Learning
Machine learning isn’t a one-size-fits-all approach; it encompasses various techniques tailored to different scenarios:
1. Supervised Learning
This approach involves training algorithms using labeled data to make predictions or decisions based on input variables.
2. Unsupervised Learning
Here, algorithms uncover patterns in unlabeled data without explicit guidance, allowing for more complex and versatile problem-solving.
3. Semi-supervised Learning
Combining elements of both supervised and unsupervised learning, this approach utilizes a mix of labeled and unlabeled data to train models effectively.
4. Reinforcement Learning
In reinforcement learning, models learn through trial and error, receiving rewards or penalties based on their actions, akin to a process of learning from experience.
Distinguishing Machine Learning, Deep Learning, and AI
While often used interchangeably, these terms carry distinct nuances:
Artificial Intelligence (AI)
AI encompasses machines’ ability to simulate human-like intelligence and behavior, aiming to replicate cognitive functions.
Machine Learning
A subset of AI, machine learning focuses on training algorithms to learn from data and make predictions or decisions without explicit programming.
Deep Learning
Deep learning, a subset of machine learning, involves training neural networks to process and analyze data, mimicking the intricate structure of the human brain.
Ethical Concerns of Machine Learning Applications
As with any transformative technology, machine learning presents ethical considerations:
1. Surveillance Concerns
While enhancing security measures, surveillance technologies fueled by machine learning raise questions about privacy and personal freedoms.
2. Transparency and Accountability
The lack of regulatory frameworks and transparency surrounding AI technologies raises concerns about data privacy and accountability.
3. Power Imbalances
The concentration of AI resources in the hands of few large companies can exacerbate inequalities and stifle competition in the market.
4. Misinformation Risks
Limitations in data quality and bias can lead to the proliferation of inaccurate information, posing risks to societal discourse and decision-making.
5. Data Privacy
The ubiquitous collection of personal data raises concerns about data privacy and security, highlighting the need for robust safeguards and regulations.
Base Delhi IP irctc Open Proxy
An open proxy can expose users to security vulnerabilities, allowing unauthorized access to sensitive information and compromising online privacy.
To mitigate these risks, individuals should avoid using open proxies and instead opt for secure solutions like ForestVPN. It encrypts your internet connection, safeguarding your data from prying eyes.
FAQ about Machine Learning Applications
1. What are the key applications of machine learning?
Machine learning finds applications in diverse domains, including personalized recommendations, fraud detection, virtual assistants, traffic prediction, and facial recognition.
2. How does machine learning differ from deep learning and AI?
Machine learning is a subset of AI focused on training algorithms to learn from data. Deep learning, in turn, is a subset of machine learning that involves training neural networks.
3. What are the ethical challenges associated with machine learning?
Ethical challenges in machine learning include concerns about surveillance, transparency, power imbalances, misinformation risks, and data privacy.
4. How does supervised learning differ from unsupervised learning?
In supervised learning, algorithms are trained using labeled data, while unsupervised learning involves uncovering patterns in unlabeled data without explicit guidance.
5. How can individuals protect their privacy in the age of machine learning?
Individuals can protect their privacy by being mindful of the data they share online, utilizing privacy-enhancing technologies like VPNs, and advocating for robust data protection regulations.